Predictive Maintenance and Its Pros & Cons
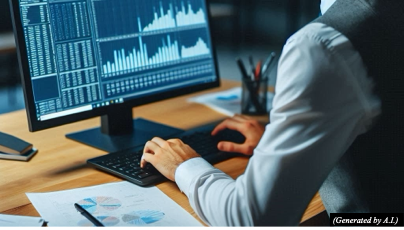
Predictive maintenance entails evaluating a machine’s condition using specific data inputs to forecast potential failures. Maintenance activities are subsequently performed based on these predictions. This methodology offers substantial advantages over traditional breakdown, corrective, and preventive maintenance by maximizing productivity and mitigating unexpected failures, which can incur significant costs.
With the advancements in technology, including the availability of connected sensors, the Internet of Things (IoT), machine learning (ML), and artificial intelligence (AI), predictive maintenance is increasingly becoming a practical solution. Sensors installed on machines can transmit real-time data regarding their condition, such as the temperature of a turbine shaft or the vibration of a bearing. By analyzing these real-time readings, abnormal conditions can be predicted before they manifest.
Consider a critical air compressor in an industrial plant. The vibration levels of the compressor can be monitored continuously. Over time, this data reveals trends, such as increasing vibration levels, which may indicate potential issues. By analyzing this data, rules can be established to predict when the vibrations will reach a critical point, potentially leading to compressor failure.
Using this predicted failure date, maintenance can be planned well in advance. This includes ordering the necessary parts, scheduling the maintenance team, and selecting a date that minimizes the impact on production. This proactive approach ensures the compressor operates efficiently and avoids costly downtime.
However, as with everything else, predictive maintenance also comes with some challenges. The data collected by all the connected sensors is huge. Also, a lot of historical data is required to train accurate models which can predict the failures with accuracy.
We’ll now evaluate the pros and cons of predictive maintenance. Like any technology, predictive maintenance also has some benefits and some shortcomings. I’ll try to assess what advantages and disadvantages it brings.
Pros / Advantages of Predictive Maintenance:
- Increased Uptime of Machines: With predictive maintenance and accurate models the failures of the machine can be anticipated in advance, and with minor corrective maintenance, breakdowns can be avoided. This increases the uptime of the machines and thus improves productivity.
- Increased Safety: Sometimes breakdowns may result in hazardous conditions for maintenance workers. A belt on a compressor may snap which may seriously hurt a maintenance worker in the vicinity. A pressure vessel might explode due to high pressure created by a malfunctioning part, which may impact all technicians/ employees in that section of the plant. With predictive maintenance such failures can be avoided by knowing when to replace the compressor belt, or when to replace the malfunctioning pressure relief valve.
- Reduced Maintenance Cost: If the maintenance planner knows the upcoming maintenance activities well in advance, she/ he can plan it very efficiently by scheduling the technicians or service providers and buying the spare parts well in advance. This advanced planning reduces the emergency personnel costs and urgent parts deliveries and saves a lot of maintenance costs.
- Reduced Failures: MTTF (mean time to failure) and MTBR (mean time between repairs) can be drastically increased with predictive maintenance by avoiding any unforeseen failures or breakdowns.
- Reduced Inventory Cost: With predictive maintenance planners can move away from preventive maintenance, which is more efficient than corrective or breakdown, but still consumes a lot of spare parts by following a set interval for maintenance. With predictive maintenance the replacement of spare parts can be optimized by having real-time feedback of the condition of the part.
Cons / disadvantages of Predictive Maintenance:
- Expensive Initial Set Up: While predictive maintenance can save a lot of maintenance costs over the long run, the initial setup can be very expensive. Currently only the very big organizations can afford it. It needs equipment upgrades, sensors enabled with IoT, network connectivity and huge data storage capabilities. Apart from this, resources with the correct skill set are also rare to find since the technology is new. If you combine all these initial costs, it will mean a substantial initial investment.
- Security: At the backbone of any predictive maintenance solution is connectivity and data, there is always a risk of security. A risk of malware or someone hacking the system to gain access to the data is ever present.
- Increased Efforts: Although the breakdown, corrective and preventive maintenance efforts go down with predictive maintenance, there is still a lot of effort required to interpret the huge amount of data and interpret it into actionable maintenance. Training the models with ML and AI also requires a lot of effort and specialized skill.
- Reduced Manpower Needs: With algorithms doing a lot of monitoring of machines, the need for planners and technicians taking rounds of the plant to take readings etc. goes down. Job loss of existing employees, which can be replaced by more efficient predictive maintenance programs and online sensors collecting data all the time.
- Dependency on Data Accuracy: To define effective and accurate predictive models which can forecast upcoming breakdowns or malfunctions accurately is of utmost importance. Historical data plays a critical role in defining models. If the data used to build predictive models is not reliable and accurate then the models will not be reliable. Lack of data is a serious challenge for implementing an effective predictive maintenance.
Conclusion:
Based on the points above I think predictive maintenance could be very helpful for the efficient upkeep of machines and reduction of overall maintenance costs. In my opinion the best approach for most industries towards predictive maintenance should be to start small, assess the results and then scale. With more advances in the technology and availability of skilled resources predictive maintenance could be the future of maintenance.
About the Author – Amit Saxena
Amit Saxena has done M.S. in Data Science, B.E. in Marine Engineering and works as an Architect in an MNC.
He has more than 22 years of experience in Enterprise Asset Management across various industries and is working towards the usage of AI and ML in improving the Maintenance Processes.